NLPIR SEMINAR Y2019#1
INTRO
In the new semester, our Lab, Web Search Mining and Security Lab, plans to hold an academic seminar every Wednesdays, and each time a keynote speaker will share understanding of papers published in recent years with you.
Arrangement
This week’s seminar is organized as follows:
1. The seminar time is 1.pm, Wed., at Zhongguancun Technology Park ,Building 5, 1306.
2. The lecturer is Baohua Zhang, the paper’s title is Curriculum Learning for Natural Answer Generation.
3. The seminar will be hosted by Zhaoyang Wang.
4. Attachment is the paper of this seminar, please download in advance.
Anyone interested in this topic is welcomed to join us. the following is the abstract for this week’s paper.
Curriculum Learning for Natural Answer Generation
Cao Liu, Shizhu He, Kang Liu, Jun Zhao
Abstract
By reason of being able to obtain natural language responses, natural answers are more favored in real-world Question Answering (QA) systems. Generative models learn to automatically generate natural answers from large-scale question answer pairs (QA-pairs). However, they are suffering from the uncontrollable and uneven quality of QA-pairs crawled from the Internet. To address this problem, we propose a curriculum learning based framework for natural answer generation (CL-NAG), which is able to take full advantage of the valuable learning data from a noisy and uneven-quality corpora. Specifically, we employ two practical measures to automatically measure the quality (complexity) of QA-pairs. Based on the measurements, CLNAG firstly utilizes simple and low-quality QApairs to learn a basic model, and then gradually learns to produce better answers with richer contents and more complete syntaxes based on more complex and higher-quality QA-pairs. In this way, all valuable information in the noisy and unevenquality corpora could be fully exploited. Experiments demonstrate that CL-NAG outperforms the state-of-the-art, which increases 6.8% and 8.7% in the accuracy for simple and complex questions, respectively.
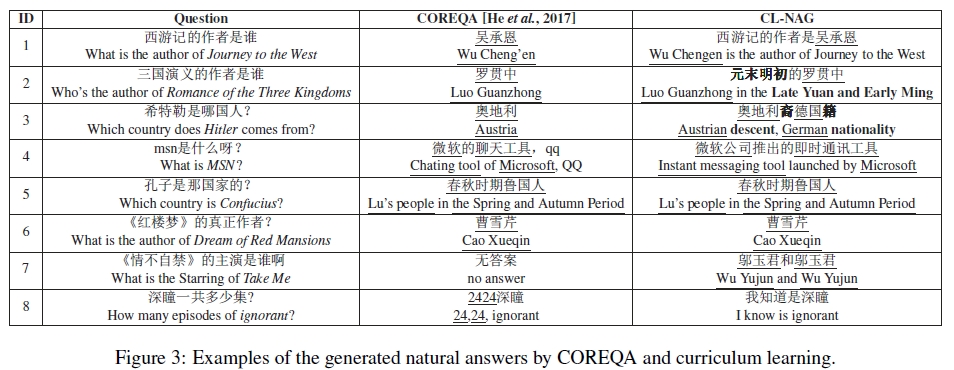